Decarbonizing Data
You are reading this Voyager letter thanks to the ceaseless efforts of the globe-spanning system of metal, fiber, electrons, photons, and software that make up today’s data centers and data transmission networks. Voyager’s partners used cloud-based services to compose this note, store research materials, analyze data, and finally publish to thousands of inboxes simultaneously. We easily forget the degree of simplification and abstraction enabled by today’s global data centers and the services built atop them. We should not. Underneath dematerialized services lives an inescapable physical network and asset base that is inextricably linked to our energy and climate system. There is real power behind our virtual worlds.
These dematerialized services, from cloud-based analytics to generative AI, will create significant economic value in the next few decades. Their influence on the energy system will be consequential. They may significantly decarbonize emissions-intensive sectors; at the same time, the scale of their own energy consumption may delay or defray systemic sustainability. The world’s data centers, network operations, and distributed computing systems already consume several percent of the world’s total power generation. Without technological intervention, this energy demand and commensurate greenhouse gas emissions could grow significantly. Today’s data centers exist in a quantum energy state, so to speak: simultaneously an agent of transformation for the better, or a driver of emissions for the worse.
Standalone data centers, which were vanishingly contributors to global energy demand two decades ago, are now significant sources of power demand and drivers of global greenhouse gas emissions. In 2021, data centers consumed between 220 and 320 terawatt-hours of electricity, about as much as the UK consumes in a year and equivalent to roughly 1% of global electricity consumption. In that same year, cryptocurrency mining consumed an estimated 140 gigawatt-hours of electricity, slightly more electricity than Norway or Sweden consume annually.
Data center electricity growth has actually been quite modest, relative to the growth in the data center workloads. The International Energy Agency estimates that non-crypto data center electricity consumption expanded between 10% and 60% from 2015 to 2021; at the same time, the number of internet users increased 60%, data center workloads increased 260%, and internet traffic increased 440%.
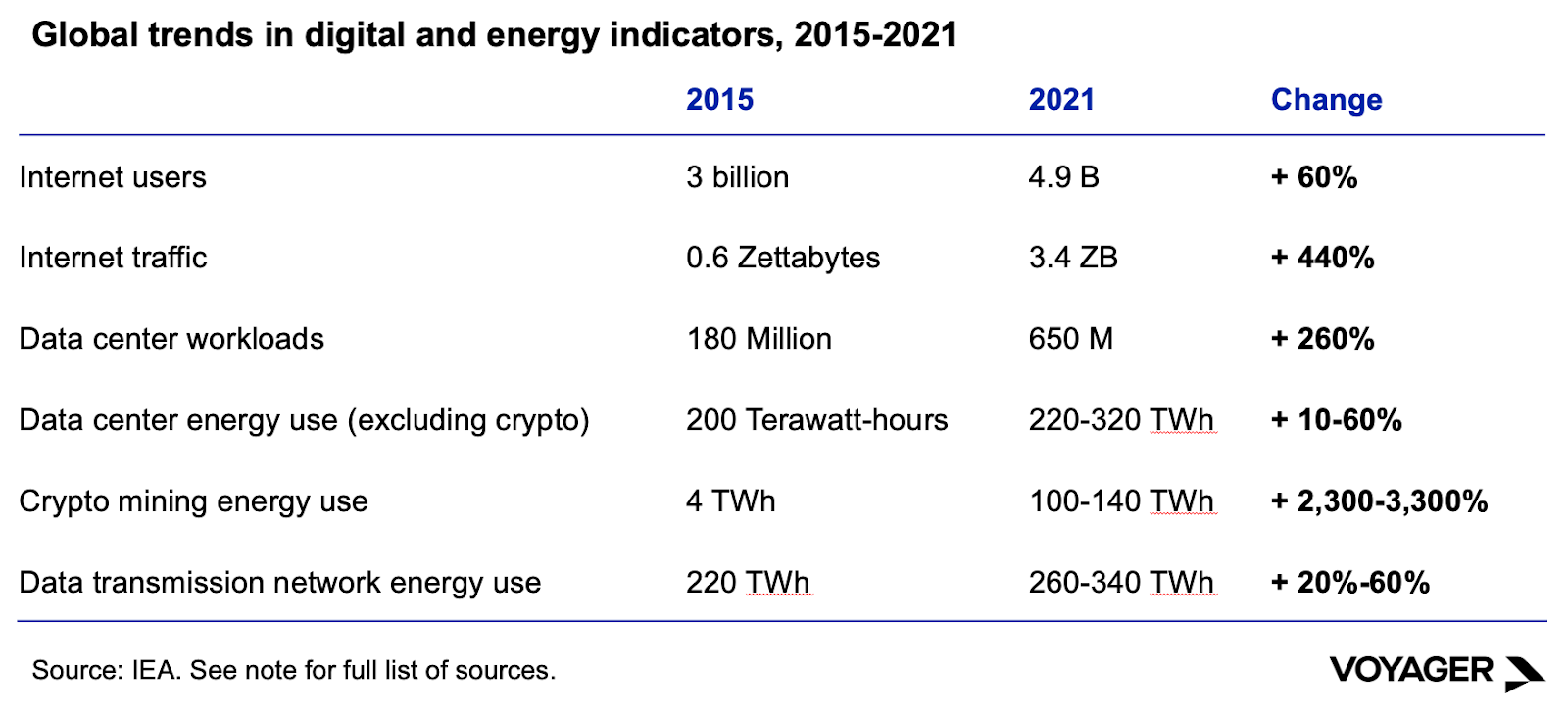
A full list of sources is here.
Renewable power purchases now match much of the new electricity demand created by the world’s largest data centers and their largest operators, which – to an extent - mitigate data centers’ environmental impact. In 2022, Amazon, Meta, Google parent company Alphabet, and Microsoft were the biggest corporate purchases of clean power; Amazon, which currently contracts just under 25 gigawatts of wind and solar, is responsible for the seventh-biggest clean power fleet globally (including power utilities).
The relatively modest growth in energy consumption relative to the growth in users, workloads, and data traffic, combined with the outsized economic benefit provided from data centers’ global scale and reach, could give the impression that data center energy is a problem with a scalar solution. Couple greater efficiency with more clean power, and data will decarbonize. It is a beguiling position – but it is misleading.
Modest energy demand growth is still growth, and not every new workload can be offset with renewable power. Data center capital expenditure is still growing at double-digit percentage points a year, and in 2022 reached nearly 240 billion dollars. Renewable power generation costs are rising thanks to inflation and higher interest rates, which complicates previously-automatic renewable power procurement strategies.
Today’s existing data center workloads might live in the cloud, but they are still highly exposed to physical disruption. A recent survey found that 45% of data center operators had experienced some form of weather-related threat to continuous operations, and that almost 9% of operators experienced an actual weather-related disruption. Climate change will make these weather impacts more frequent, and the threats of disruption more severe.
And beyond operational challenges, an energy and computational giant appears on the horizon: artificial intelligence.
AI-related compute demand is, appropriately enough, becoming general in scope. In its latest earnings call, Microsoft’s CFO Amy Hood said that “we will continue to invest in our cloud infrastructure, particularly AI-related spend as we scale with the growing demand, driven by customer transformation.” One semiconductor executive says that AI’s energy consumption is a looming energy crisis. That rhetoric may be extreme, but the concern is real.
Generative AI, large language model training, and the expansion of the energy-intensive backbones they require create new imperatives for data center energy efficiency, a finer-grained understanding of data’s energy and climate impacts, and development of new technology.
Voyager considers this new era through three lenses: the history of data center efficiency to date, custom compute and capabilities, and new efficiency frontiers.
Fiber, Wires, Wind, Sun, and PUE
Electronic computation has been an energy-intensive process since its inception. ENIAC, the world’s first large-scale electronic computer, consumed 174 kilowatts of power in the mid-1940s, or about 70% of what today’s Tesla Superchargers can provide. The Cray-1 supercomputer debuted in the 1970s with orders of magnitude more capability with less power consumption – about 115 kilowatts. The current fastest supercomputer, HPE’s Frontier, draws 22,703 kilowatts of power. This sort of compute is, and always has been, an edge case. Today’s hyperscale data centers provide much less novel services at much greater scale. Getting to scale, though, began very modestly.
Google’s first data center was not custom built in an optimal location; it was not powered by solar or wind. It was a seven foot by four foot (2.5 square meter) cage inside one of the first co-location facilities in Silicon Valley, filled with “about 30 PCs on shelves”. The entire facility, such as it was, could fit on a one-page order sheet.
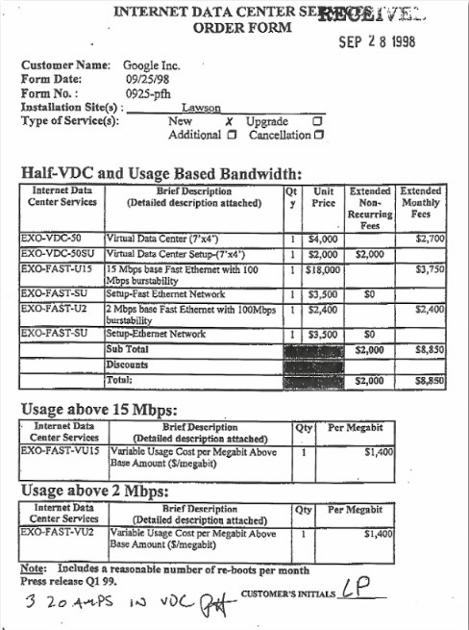
But for Google, and its data-intensive peers, it was soon obvious that urban co-location was expensive. Space was scarce, and power was expensive. Moving past those constraints meant looking beyond Silicon Valley.
In the late 1990s, the Bonneville Power Administration, which operates a network of large hydroelectricity facilities and long-distance bulk transmission lines in the US Northwest, began stringing fiber optic cable alongside its electrical network. That fiber was originally for its own internal communications, but the BPA’s next step will be familiar to close observers of technology development. While not remotely a technology company, the BPA’s fiber efforts placed it in familiar tech territory, of very low marginal cost. In the words of internet infrastructure chronicler Andrew Blum, the BPA quickly realized that it was “only incrementally more expensive to install extra fiber – far more, in fact, than they needed for the company’s own use”.
That extra fiber might have started dark, but it did not stay that way. Not only was the bandwidth waiting to be filled, the fiber literally mapped to an extensive, low-cost, reliable network of mostly low-carbon power generation. Not only that, it was in a region with a forgiving climate for data centers: low humidity, and (at least at the time) few extremely hot periods. In addition, the regional grid was built to accommodate large industrial electricity loads, historically for aluminum smelting.
Bonneville Power Transmission System and Federal Dams
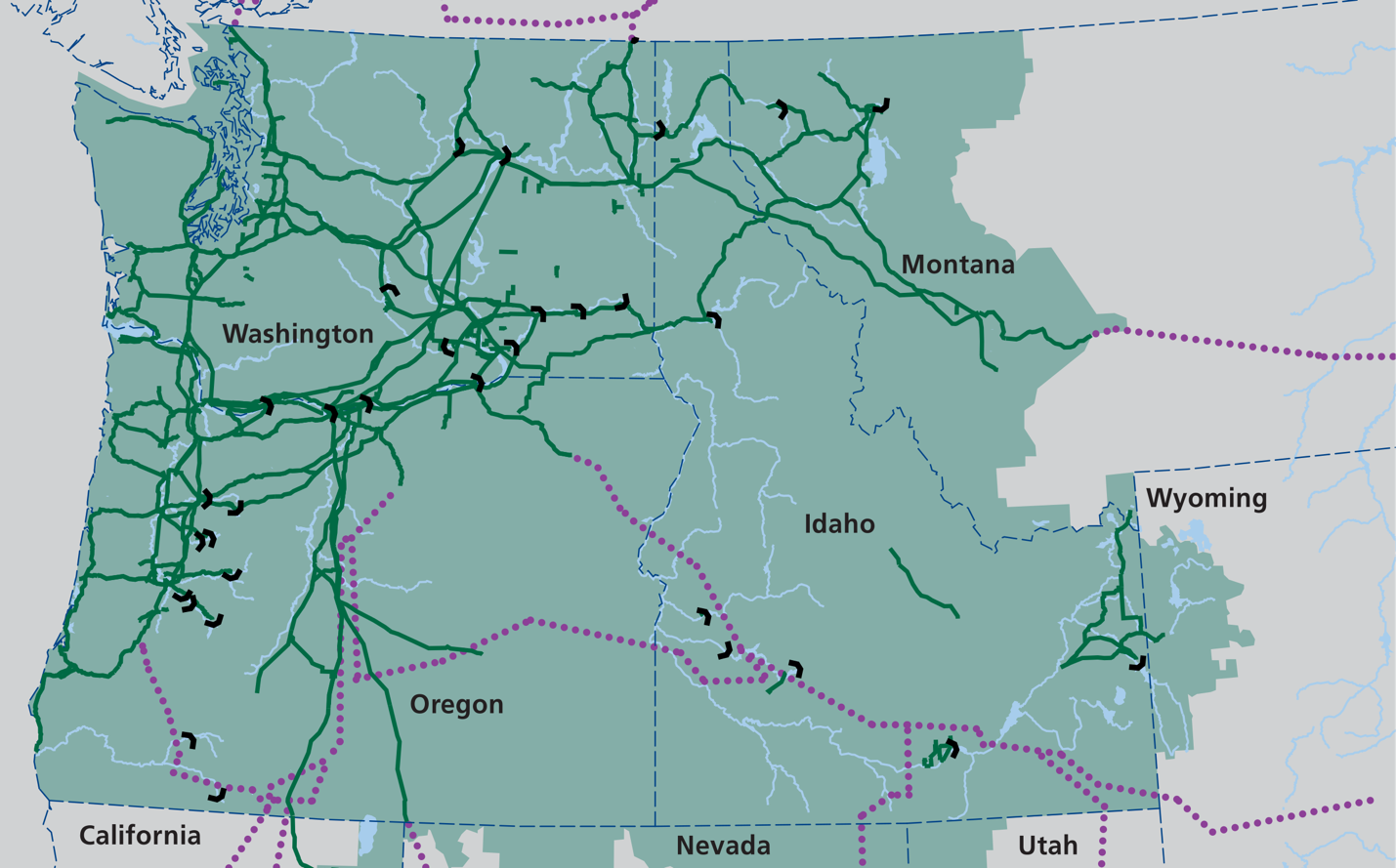
Source: Bonneville Power Administration, fiscal year 2019.
In 2004, the small Oregon town of The Dalles had an unusual visitor. Located on the Columbia River, and the former site of an aluminum smelter consuming 85 megawatts of power, the town hosted a now-familiar climate tech investor with a purposefully vague remit. As Blum tells it:
A man named Chris Sacca, representing a company with the suspiciously generic name of “Design LLC”, showed up in The Dalles looking for shovel-ready sites in “enterprise zones,” where tax breaks and other incentives were offered to encourage businesses to locate there. He was young, sloppily dressed, and interested in such astronomical quantities of power that a nearby town had suspected him of being a terrorist and called the Department of Homeland Security.
Sacca was not, it is now obvious, a terrorist. He was one of the first of his kind, however: a prospector of sorts, after power. The ensuing two decades of data center build-out would lead to ever-larger facilities and also, ever-more-efficient facilities. As Google’s first cage in Silicon Valley would suggest, there were many operational aspects of early data centers in need of optimizing. There were significant electrical loads for lighting, for cooling, for powering fluid pumps, and generally for making an enclosed space habitable for both humans and machines.
As data centers became larger, those additional power loads could become a smaller segment of total power consumed. Hyperscale operators began designing their own efficient servers, and eventually their own silicon. Engineers laid out servers with ‘hot aisles’ and ‘cold aisles’ as if data centers were supermarkets, concentrating hardware with higher heat tolerances. Interior ambient temperatures were allowed to run hotter than a person might prefer, but within machine parameters – with the tradeoff being lighter-weight clothing for site staff. Data centers became almost organic, oriented around circulating and respiring, sensing and acting. The resulting structures were also more efficient. A 2018-2020 Microsoft study found that the largest data centers could be as much as 93% more energy efficient than on-premise or self-operated enterprise data centers.
The best measure of that increasing efficiency is known as power usage effectiveness, a measure developed in 2016 by the Green Grid consortium. PUE is a ratio measuring how much of a data center’s power usage is for compute versus cooling, air handling, and lighting.

An ideal PUE would be 1.0 – that is, every bit of energy used in a data center is used by and for compute. That is not possible, of course, but what has been possible in the past 15 years is the compression of PUE to its logical minimum. Google data center PUE was at 1.08 at the end of 2022, down from 1.23 in 2008.
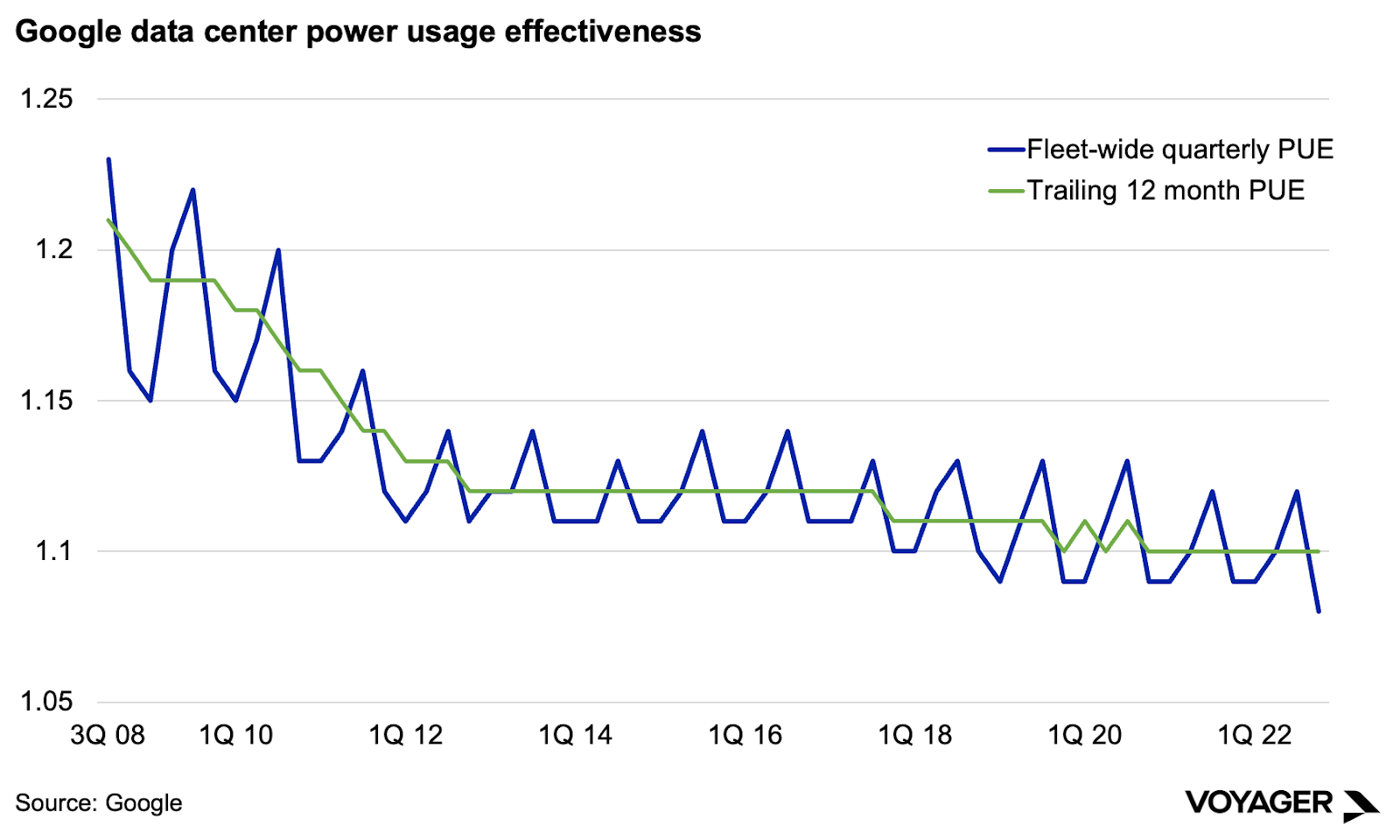
PUE is still seasonal, and that is thanks to a data center concentration in the northern hemisphere, and a resulting summer spike in cooling load, It also seems to have reached an asymptotic minimum. It is hard to imagine it moving much lower using existing technology and approaches.
This inevitable plateau is why renewable power procurement is so important to data center decarbonization. Changing today’s energy inputs – and innovating on tomorrow’s inputs – will enable data centers to operate as close to climatically neutral as possible. True 24/7 zero-carbon power is a priority for the world’s biggest data center operators, and it has not been realized yet by most.
But data center PUE reaching its asymptotic minimum is also why it is important for operators and technology developers to move beyond procurement strategies to decarbonize data. Tomorrow’s data operations will need more than a buying strategy; they need imagination, and re-imagination.
Customizing Compute and Creatively Managing Demand
Changing compute’s energy demand in the future begins with changing compute itself. We have already experienced several hardware innovations which optimize specific processors for specific tasks. Google’s first data centers were built using general purpose CPUs; today’s hyperscale compute, be it for large language models or graphics-intensive work, runs on chips tailored for specific workflows. The world’s biggest tech companies are also designing custom chips for running AI models.
There is more to do. Custom chips to run specialized computing tasks are enabling an explosion of innovation in machine learning. In doing so they follow a useful trajectory. Customized chips enabled high-resolution graphics processing that in turn enabled Pixar and Fortnite. Those chips, pushed to their extremes in machine learning applications, then led to new specialization for AI applications, as even a casual observer of NVIDIA will have noticed. The backbone of computing tasks that data centers run, however – the data analytics that powers core computing from databases and webpages – does not run on specialized chips. These tasks run on general-purpose CPUs, borrowed from other applications.
However, new, custom chips could dramatically improve the performance and massively shrink the energy demand of data analytics processing – potentially by up to 90%. Voyager portfolio company Intensivate is designing and building these chips. It promises to not only slash energy use and related emissions in data centers, but free up space and power capacity to run machine learning tasks in the same building footprint, saving additional costs on infrastructure, cooling, and energy.
The next steps in data center and compute efficiency will require new physics. The prime mover of six decades of improvements in computing hardware is the rapid and persistent doubling in transistor density, which many will know via the shorthand of its eponymous observer: Moore’s Law. We are reaching the limits of Moore’s Law using existing manufacturing techniques. Current feature sizes have reached as small as 3 nanometers, only a few times bigger than the silicon atoms from which they are made. Smaller features will require new, or radically different, paradigms of computing hardware: perhaps not only manufacturing, but also software and functions. Possible successor technologies that could extend or surpass Moore’s Law include optical computing and quantum computing, among others. These technologies, should they ever be widely commercialized, may excel at highly specialized computing tasks, and as those specialized tasks grow in scope, they could potentially replace much of today’s compute as well.
A final step in further refining efficiency will be to creatively consider the nature of compute demand. Hyperscale data center operators already shift compute loads across sites and regions in order to capture the lowest-cost and lowest-emissions power generation sources. The future will require more than just shifting in time and space; it will reward those who design their compute needs around knowable, anticipated periods in which energy is widely available, zero-carbon, and low-cost, free, or even negatively priced.
Voyager notes that cryptocurrency mining is one approach that meets these parameters. As noted above, it also consumes a Scandinavian country’s worth of power in the process. In the US, cryptocurrency mining may consume more power than all residential illumination. Crypto mining, with its massive and concentrated demand, can be both the cause of and solution to significant energy problems in strained grids. That said, the imperative, from Voyager’s perspective, should be climate-first. That is: climate benefits are paramount in a company’s cryptocurrency value proposition, and not a justification.
Other solutions can be just that – solutions first and foremost. Computing loads can be designed with time-shifting as an operational feature, not a bug. Businesses and business models can be built around absorbing variability rather than responding to it. Ideal cases will be able to operate in a truly neutral fashion: using otherwise-curtailed renewable power to create a value-added product using optimized existing infrastructure.
The Efficient Frontier
There is an Easter Egg, of sorts, hidden in this letter’s first table. The number of internet users, the total volume of data center workloads, and data flows through global communications networks are all well known. A close look at the table above, however, shows that data center and data transport energy consumption live in the realm of estimates, and broad estimates at that. Improving our understanding of data’s energy consumption is an essential part of improving operations at every level.
The world would be fortunate to discover that data’s energy consumption is at the low end of its ranges, rather than the high end. That low end implies a greater store of paid-in capital in the form of processes and procedures for reducing data’s energy demand.
But if we are at the high end, a reasonable assumption given how much compute still takes place in enterprise data centers and/or on-premise, then the world’s data-energy optimizers have a bigger challenge ahead of them. We should hope that all new model operators are as transparent as Hugging Face, which published the energy consumption of its 176 billion-parameter large language model, BLOOM. Bloom’s final training required more than a million GPU hours and consumed more than 433 megawatt-hours of electricity. That power consumption resulted in almost 25 tons of CO2 emissions; the embedded emissions in its equipment as well as the non-computational energy consumption required for operations doubled that figure.
Hugging Face’s transparency hints at the challenge ahead: going deep into operational energy consumption requires significant transparency. It also hints at the bigger opportunity ahead. There will always be more room to mesh the gears of process innovation ever-finer. And, there will be more space for invention and reimagination throughout hardware, software, operations, and manufacturing as well.
Today’s digital infrastructure system provides more societal value than ever before, with a greater climate footprint than ever before. Increasingly advanced computing is positioned to bring major advances in medicine, science, and business. It can accelerate the design and deployment of foundational climate technologies. As we expand our use of computing power, we can embrace its potential not just to change other systems, but to change itself.